Catalogue Search | MBRL
نتائج البحث
MBRLSearchResults
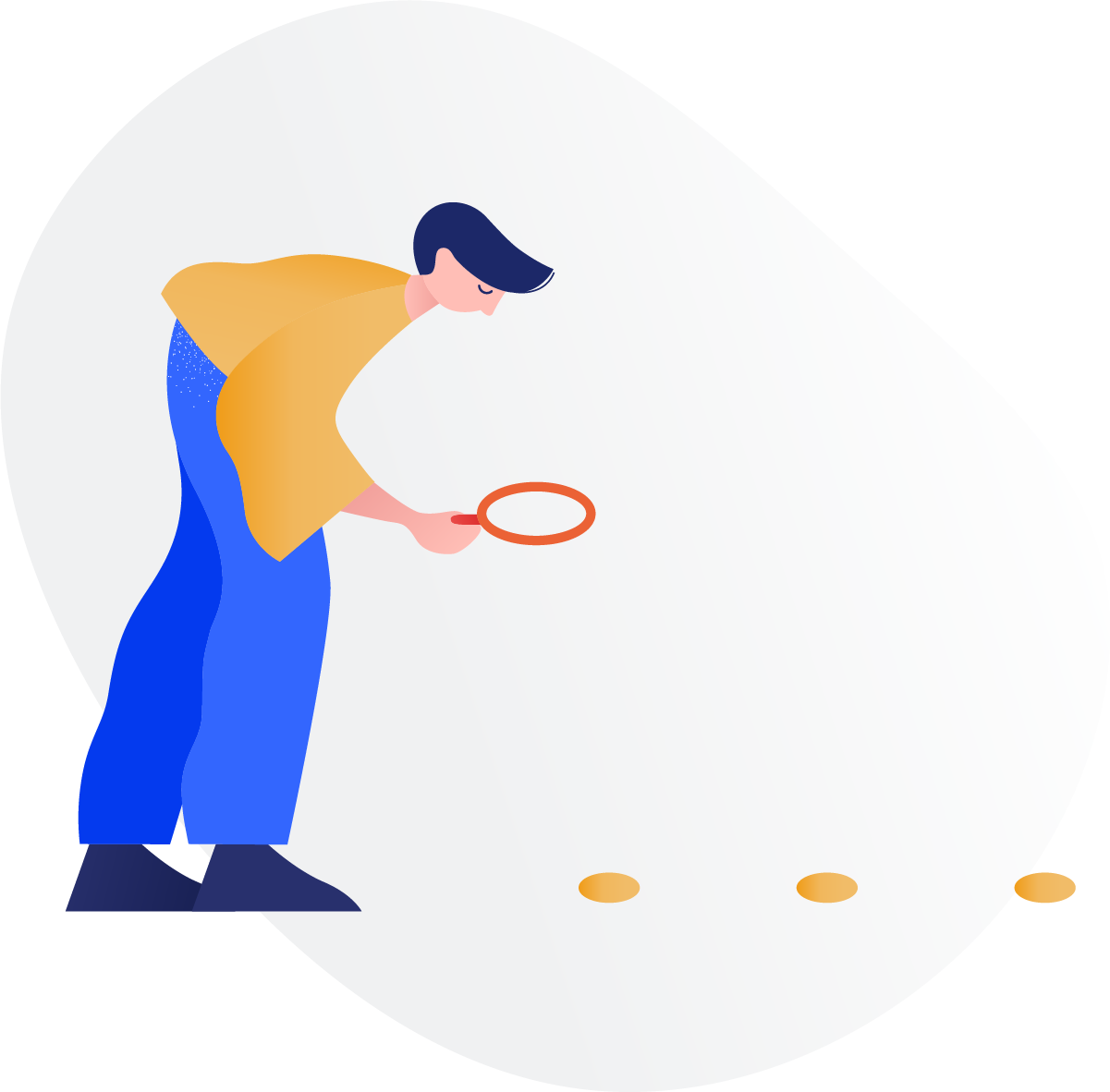
وجه الفتاة! هناك خطأ ما.
أثناء محاولة إضافة العنوان إلى الرف ، حدث خطأ ما :( يرجى إعادة المحاولة لاحقًا!
-
الضبطالضبط
-
مُحَكَّمةمُحَكَّمة
-
نوع العنصرنوع العنصر
-
الموضوعالموضوع
-
السنةمن:-إلى:
-
المزيد من المرشحاتالمزيد من المرشحاتالمصدراللغة
منجز
مرشحات
إعادة تعيين
12,638
نتائج ل
"lung X-ray"
صنف حسب:
Machine Learning Algorithm for Trend Analysis in Short term Forecasting of COVID-19 using Lung X-ray Images
بواسطة
George, G.Victo Sudha
,
Kirubakaran, D.
,
Sujatha, K
في
Convolutional Neural Networks
,
COVID-19
,
Forecasting efficiency
2023
Abstract With the development of medical technology, the diagnosis of lung diseases relies more on the determination of medical images. With increasingly huge data, a powerful data processing model is urgently needed to provide favorable support for this field. The goal of this study is to develop a computer-assisted method to identify COVID-19 from X-ray pictures of the lungs at the very beginning of the disease. The architecture is implemented as a software system on a computer that can assist in the affordable and accurate early identification of cardiac illness. The performance of CNN architecture is best among all other classification algorithms to detect COVID-9 from Lung X-ray images. The datasets consist of COVID-19 established cases for 4 weeks which included the X-ray images of the chest. Then the distribution of the data was examined according to the statistical distribution. For this prediction, time series models are used for forecasting the pandemic situation. The performances of the methods were compared according to the MSE metric and it was seen that the Convolutional Neural Networks (CNN) achieved the optimal trend pattern.
Journal Article
Lung X-ray Segmentation using Deep Convolutional Neural Networks on Contrast-Enhanced Binarized Images
بواسطة
Ruan, Shanq-Jang
,
Peng, Yan-Tsung
,
Chen, Hsin-Jui
في
deep convolutional neural networks
,
histogram equalization
,
image binarization
2020
Automatically locating the lung regions effectively and efficiently in digital chest X-ray (CXR) images is important in computer-aided diagnosis. In this paper, we propose an adaptive pre-processing approach for segmenting the lung regions from CXR images using convolutional neural networks-based (CNN-based) architectures. It is comprised of three steps. First, a contrast enhancement method specifically designed for CXR images is adopted. Second, adaptive image binarization is applied to CXR images to separate the image foreground and background. Third, CNN-based architectures are trained on the binarized images for image segmentation. The experimental results show that the proposed pre-processing approach is applicable and effective to various CNN-based architectures and can achieve comparable segmentation accuracy to that of state-of-the-art methods while greatly expediting the model training by up to 20.74 % and reducing storage space for CRX image datasets by down to 94.6 % on average.
Journal Article
Enhancing medical image segmentation with a multi-transformer U-Net
بواسطة
Jin, Weishou
,
Yue, Xuebin
,
Dan, Yongping
في
Computational Science
,
COVID-19
,
COVID-19 - diagnostic imaging
2024
Various segmentation networks based on Swin Transformer have shown promise in medical segmentation tasks. Nonetheless, challenges such as lower accuracy and slower training convergence have persisted. To tackle these issues, we introduce a novel approach that combines the Swin Transformer and Deformable Transformer to enhance overall model performance. We leverage the Swin Transformer's window attention mechanism to capture local feature information and employ the Deformable Transformer to adjust sampling positions dynamically, accelerating model convergence and aligning it more closely with object shapes and sizes. By amalgamating both Transformer modules and incorporating additional skip connections to minimize information loss, our proposed model excels at rapidly and accurately segmenting CT or X-ray lung images. Experimental results demonstrate the remarkable, showcasing the significant prowess of our model. It surpasses the performance of the standalone Swin Transformer's Swin Unet and converges more rapidly under identical conditions, yielding accuracy improvements of 0.7% (resulting in 88.18%) and 2.7% (resulting in 98.01%) on the COVID-19 CT scan lesion segmentation dataset and Chest X-ray Masks and Labels dataset, respectively. This advancement has the potential to aid medical practitioners in early diagnosis and treatment decision-making.
Journal Article
Classification and Predictions of Lung Diseases from Chest X-rays Using MobileNet V2
2021
Featured Application: The method presented in this paper can be applied in medical computer systems for supporting medical diagnosis.Abstract: Thoracic radiography (chest X-ray) is an inexpensive but effective and widely used medical imaging procedure. However, a lack of qualified radiologists severely limits the applicability of the technique. Even current Deep Learning-based approaches often require strong supervision, e.g., annotated bounding boxes, to train such systems, which is impossible to harvest on a large scale. In this work, we proposed the classification and prediction of lung pathologies of frontal thoracic X-rays using a modified model MobileNet V2. We considered using transfer learning with metadata leverage. We used the NIH Chest-Xray-14 database, and we did a comparison of performance of our approach to other state-of-the-art methods for pathology classification. The main comparison was by Area under the Receiver Operating Characteristic Curve (AUC) statistics and analyzed the differences between classifiers. Overall, we notice a considerable spread in the achieved result with an average AUC of 0.811 and an accuracy above 90%. We conclude that resampling the dataset gives a huge improvement to the model performance. In this work, we intended to create a model that is capable of being trained, and modified devices with low computing power because they can be implemented into smaller IoT devices.
Journal Article
Improving Respiratory Infection Diagnosis with Deep Learning and Combinatorial Fusion: A Two-Stage Approach Using Chest X-ray Imaging
بواسطة
Kumar, Rahul
,
Chang, Chun-Yung
,
Shiue, Yow-Ling
في
Algorithms
,
Artificial intelligence
,
combinatorial fusion
2024
The challenges of respiratory infections persist as a global health crisis, placing substantial stress on healthcare infrastructures and necessitating ongoing investigation into efficacious treatment modalities. The persistent challenge of respiratory infections, including COVID-19, underscores the critical need for enhanced diagnostic methodologies to support early treatment interventions. This study introduces an innovative two-stage data analytics framework that leverages deep learning algorithms through a strategic combinatorial fusion technique, aimed at refining the accuracy of early-stage diagnosis of such infections. Utilizing a comprehensive dataset compiled from publicly available lung X-ray images, the research employs advanced pre-trained deep learning models to navigate the complexities of disease classification, addressing inherent data imbalances through methodical validation processes. The core contribution of this work lies in its novel application of combinatorial fusion, integrating select models to significantly elevate diagnostic precision. This approach not only showcases the adaptability and strength of deep learning in navigating the intricacies of medical imaging but also marks a significant step forward in the utilization of artificial intelligence to improve outcomes in healthcare diagnostics. The study's findings illuminate the path toward leveraging technological advancements in enhancing diagnostic accuracies, ultimately contributing to the timely and effective treatment of respiratory diseases.
Journal Article
Recognition of corona virus disease (COVID-19) using deep learning network
بواسطة
Abutiheen, Zinah Abdulridha
,
Abdulmunem, Ashwan A.
,
Aleqabie, Hiba J.
في
Algorithms
,
Computer viruses
,
Coronaviruses
2021
Corona virus disease (COVID-19) has an incredible influence in the last few months. It causes thousands of deaths in round the world. This make a rapid research movement to deal with this new virus. As a computer science, many technical researches have been done to tackle with it by using image processing algorithms. In this work, we introduce a method based on deep learning networks to classify COVID-19 based on x-ray images. Our results are encouraging to rely on to classify the infected people from the normal. We conduct our experiments on recent dataset, Kaggle dataset of COVID-19 X-ray images and using ResNet50 deep learning network with 5 and 10 folds cross validation. The experiments results show that 5 folds gives effective results than 10 folds with accuracy rate 97.28%.
Journal Article
A Novel Fuzzy DBNet for Medical Image Segmentation
بواسطة
Huang, Pei-Chen
,
Chang, Sheng-Wen
,
Chin, Chiun-Li
في
Algorithms
,
anteroposterior
,
butterfly network
2023
When doctors are fatigued, they often make diagnostic errors. Similarly, pharmacists may also make mistakes in dispensing medication. Therefore, object segmentation plays a vital role in many healthcare-related areas, such as symptom analysis in biomedical imaging and drug classification. However, many traditional deep-learning algorithms use a single view of an image for segmentation or classification. When the image is blurry or incomplete, these algorithms fail to segment the pathological area or the shape of the drugs accurately, which can then affect subsequent treatment plans. Consequently, we propose the Fuzzy DBNet, which combines the dual butterfly network and the fuzzy ASPP in a deep-learning network and processes images from both sides of an object simultaneously. Our experiments used multi-category pill and lung X-ray datasets for training. The average Dice coefficient of our proposed model reached 95.05% in multi-pill segmentation and 97.05% in lung segmentation. The results showed that our proposed model outperformed other state-of-the-art networks in both applications, demonstrating that our model can use multiple views of an image to obtain image segmentation or identification.
Journal Article
Routine lung ultrasound to detect postoperative pulmonary complications following major abdominal surgery: a prospective observational feasibility study
بواسطة
Daams, F.
,
van der Peet, D. L.
,
Bronkhorst, E. M.
في
Abdomen
,
Abdominal surgery
,
Atelectasis
2019
Background
Postoperative pulmonary complications after major abdominal surgery are associated with adverse outcome. The diagnostic accuracy of chest X-rays (CXR) to detect pulmonary disorders is limited. Alternatively, lung ultrasound (LUS) is an established evidence-based point-of-care diagnostic modality which outperforms CXR in critical care. However, its feasibility and diagnostic ability for postoperative pulmonary complications following abdominal surgery are unknown. In this prospective observational feasibility study, we included consecutive patients undergoing major abdominal surgery with an intermediate or high risk developing postoperative pulmonary complications according to the Assess Respiratory risk In Surgical patients in CATalonia (ARISCAT) score. LUS was routinely performed on postoperative days 0–3 by a researcher blinded for CXR or other clinical findings. Then, reports were drawn up for LUS concerning feasibility and detection rates of postoperative pulmonary complications. CXRs were performed on demand according to daily clinical practice. Subsequently, we compared LUS and CXR findings.
Results
A total of 98 consecutive patients with an ARISCAT score of 41 (34–49) were included in the study. LUS was feasible in all patients. In 94 (95%) patients, LUS detected one or more postoperative pulmonary complications during the first four postoperative days. On day 0, LUS detected 31 out of 43 patients (72.1%) with one or more postoperative pulmonary complications, compared to 13 out of 36 patients (36.1%) with 1 or more postoperative pulmonary complications detected with CXR RR 2.0 (95 CI [1.24–3.20]) (
p
= 0.004). The number of discordant observations between both modalities was high for atelectasis 23 (43%) and pleural effusion 29 (54%), but not for pneumothorax, respiratory infection and pulmonary edema 8 (15%), 3 (5%), and 5 (9%), respectively.
Conclusions
This study shows that LUS is highly feasible and frequently detects postoperative pulmonary complications after major abdominal surgery. Discordant observations in atelectasis and pleural effusions for LUS and CXR can be explained by a superior diagnostic ability of LUS in detecting these conditions. The effects of LUS as primary imaging modality on patient outcome should be evaluated in future studies.
Journal Article
The Effect of False-Positive Results on Subsequent Participation in Chest X-ray Screening for Lung Cancer
بواسطة
Sato, Akira
,
Hamada, Shota
,
Kawakami, Koji
في
Background radiation
,
Cancer
,
Cancer screening
2016
Background: High attendance rates and regular participation in disease screening programs are important contributors to program effectiveness. The objective of this study was to examine the effects of an initial false-positive result in chest X-ray screening for lung cancer on subsequent screening participation. Methods: This historical cohort study analyzed individuals who first participated in a lung cancer screening program conducted by Yokohama City between April 2007 and March 2011, and these participants were retrospectively tracked until March 2013. Subsequent screening participation was compared between participants with false-positive results and those with negative results in evaluation periods between 365 (for the primary outcome) and 730 days. The association of screening results with subsequent participation was evaluated using a generalized linear regression model, with adjustment for characteristics of patients and screening. Results: The proportions of subsequent screening participation within 365 days were 12.9% in 3132 participants with false-positive results and 6.7% in 15 737 participants with negative results. Although the differences in attendance rates were reduced with longer cutoffs, participants with false-positive results were consistently more likely to attend subsequent screening than patients with negative results (P < 0.01). The predictors of subsequent screening participation were false-positive results (risk ratio [RR] 1.72; 95% confidence interval [CI], 1.54-1.92), older age (RR 1.17; 95% CI, 1.11-1.23), male sex (RR 1.46; 95% CI, 1.29-1.64), being a current smoker (RR 0.80; 95% CI, 0.69-0.93), current employment (RR 0.79; 95% CI, 0.70-0.90), and being screened at a hospital cancer center (vs public health centers; RR 1.36; 95% CI, 1.15-1.60). Conclusions: Our findings indicated that subsequent participation in lung cancer screening was more likely among participants with false-positive results in an initial screening than patients with negative results.
Journal Article
American Cancer Society lung cancer screening guidelines
بواسطة
Wolf, Andrew MD
,
Smith, Robert A
,
Colditz, Graham A
في
Lung cancer
,
Medical screening
,
Medical treatment
2013
Findings from the National Cancer Institute's National Lung Screening Trial established that lung cancer mortality in specific high-risk groups can be reduced by annual screening with low-dose computed tomography. These findings indicate that the adoption of lung cancer screening could save many lives. Based on the results of the National Lung Screening Trial, the American Cancer Society is issuing an initial guideline for lung cancer screening. This guideline recommends that clinicians with access to high-volume, high-quality lung cancer screening and treatment centers should initiate a discussion about screening with apparently healthy patients aged 55 years to 74 years who have at least a 30-pack-year smoking history and who currently smoke or have quit within the past 15 years. A process of informed and shared decision-making with a clinician related to the potential benefits, limitations, and harms associated with screening for lung cancer with low-dose computed tomography should occur before any decision is made to initiate lung cancer screening. Smoking cessation counseling remains a high priority for clinical attention in discussions with current smokers, who should be informed of their continuing risk of lung cancer. Screening should not be viewed as an alternative to smoking cessation. [PUBLICATION ABSTRACT]
Journal Article